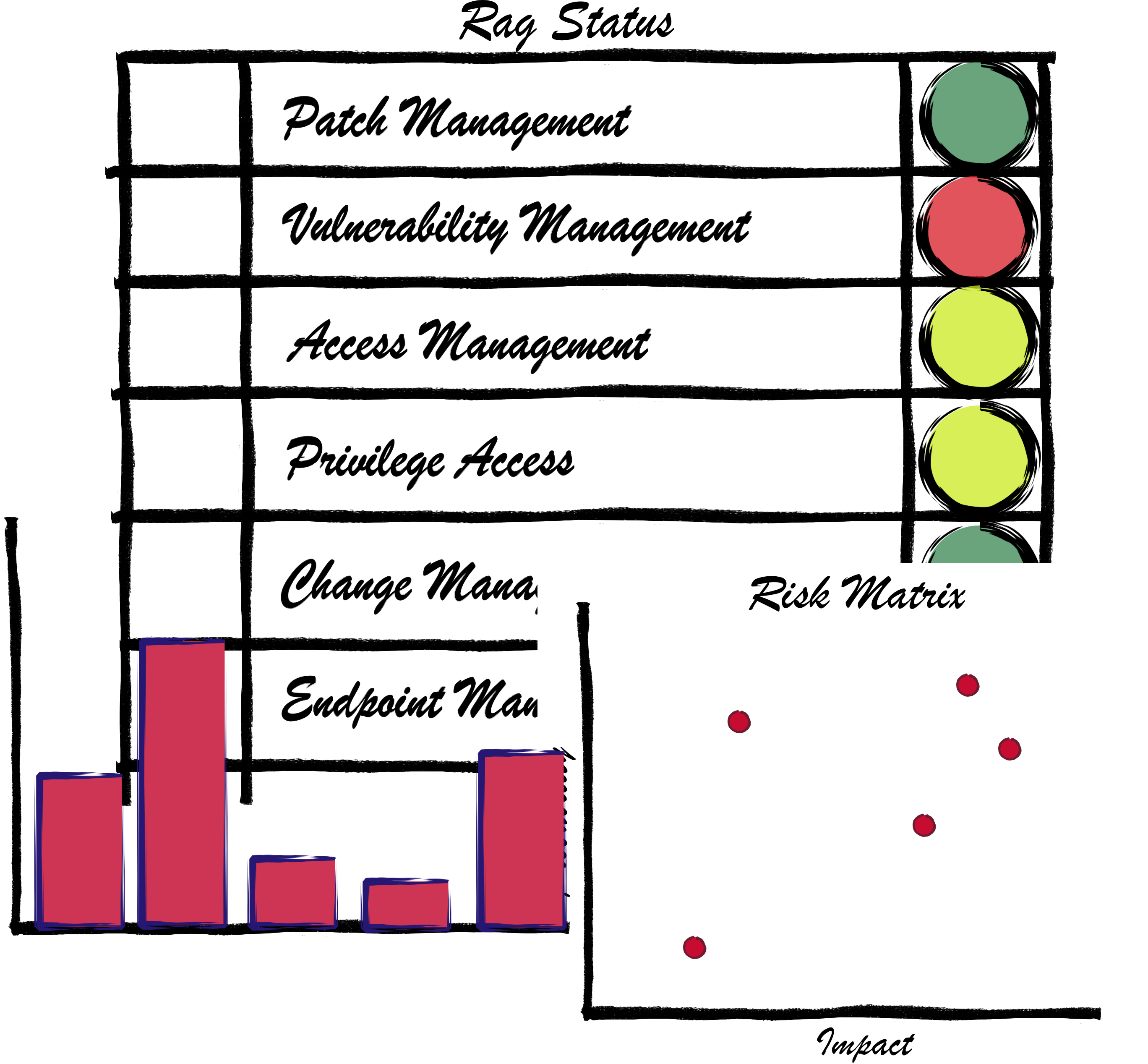
I often find myself in minor disagreements with security practitioners who want to develop quantitative (as opposed to qualitative) risk frameworks for Information Security. I tend to stand my ground on this one if only because I too thought that way many years ago and have discovered the hard way why it is infeasible. To understand why the whole idea is misguided you first need to have an appreciation for both types of risk analysis.
Information Security risk analysis has traditionally been qualitative. Most systems I have seen work on the traffic light principle, and generate a RAG status (standing for Red, Amber and Green). The data used to build the RAG status is qualitative. That is to say it is an assessment by a person and not empirically derived from data. Some practitioners will have said that given one or more factors, there is a low, medium or high risk in a particular area. Often there is some pseudo computation where impact is multiplied by probability, but the basis is always an assessment, most often a guess by some security risk person.
Some of these risk systems can give the impression of being scientific in nature. Complexity is layered upon complexity and more and more factors across a wider and wider scope are used. The issue is that if the raw data is open to interpretation then the added complexity just magnifies the uncertainty to produce more and more uncertain or inaccurate results. Added to this, the lack of certain data also increases the pointlessness of this approach. If you have a risk framework which failed to take account of your patch status for instance or perhaps it doesn’t consider the nature of the business you are in or even the police system developing in your country which is increasing hacktivism. I think it is the realisation that qualitative risk management for Information Security is so flawed that drives some practitioners to a more quantitative approach. To see why this is doomed let’s consider where quantitative risk analytics is regularly used.
Two good examples of quantitative risk analytics are financial services credit and market risk analytics and in IT operational risk. Both of these approaches work on a frequentist or statistical approach to risk. Because we see many events – either price movements for financial services or something like disk failures for IT – we are able to build up a picture of the components of a risk event. Financial services use a VAR, or Value at Risk, approach which models the risk to an organisation of the value of assets moving outside of an acceptable range. Similarly for IT, disks tend to be in RAID arrays so small disk failures don’t bring down a major application but the small failures can be used to predict the probability of a major failure – often being caused by random combinations of small failures. Both of these risk analytic systems are doing the same thing; they are looking at the frequency of measured small events and using that to predict how the risk is developing overall. In financial services they may see a trend of movements which may drive managers to adjust trading behaviour or, in IT, a rise in disk failures may indicate a problem which can be addressed by a change in maintenance schedules or in high availability or disaster recovery planning. So why can’t this approach work for Information Security then? We seem to have lots of quantitative data we could feed into a system?
There are two reasons why this approach cannot be used in our industry. The first one is the nature of the data we collect with reference to the threat and therefore the risk we face. In both of the examples above, there is a direct relationship between the events we see and the probability of the impact we wish to avoid. An increase in disk failures WILL result in a higher probability of a system failure and an increase in volatility of an asset WILL increase the risk of exceeding financial trading thresholds. For Information Security this is not the case as the small events do not correlate with the risk impacts directly. You need someone attacking you to create the impact, not simply more vulnerabilities or worse patching. The nature of the layers defined also makes this correlation harder to identify as one preventative control may create a vulnerability in another. And, of course, the data collected is often incomplete and in other ways not suitable to this task.
The second reason that this approach is doomed is also a problem for quantitative risk management in other areas too but is actually an absolute killer for Information Security. The major financial credit crunch earlier this century was missed by risk management systems because it was triggered by what is known as a black swan event. A set of circumstances which the frequentist approach rates as so unlikely as to be virtually impossible yet never-the-less can and does happen. In the financial services example, VaR produces a probability distribution which only considers values within 3 standard deviations of normal. Anything outside of that is so unlikely as to be ignored; yet the circumstances observed were far beyond that 3 standard deviation limit. Similarly, in IT risk management it is highly unlikely that several disks in one raid array will all fail together but it does happen.
Why this is a bigger problem for Information Security Risk Management is that a security breach is itself a black swan event. The one thing that a frequentist approach cannot do is predict the very thing you are interested in measuring. The whole basis for a quantitative approach to security risk management is flawed because you are effectively trying to predict the un-predictable. Predicting a security breach from the data you have is like predicting the state of the Stock Exchange this time next month. Anything you do, no matter how good it looks, is actually nothing more than a guess and all of your clever maths work is just obscuring that fact.
Yes there is still value is performing risk assessments and yes, you should hire the best risk practitioners you can because every risk assessment will be both a measure of the risk and of their ability to understand it, but their job is to help you identify where you should focus your efforts. Don’t get cross with them when they don’t predict your breach and don’t put too much belief into those RAG status charts. It is a guide, or a simplification, not a prediction. And when they suggest a more quantitative approach – ask them if they can also help with your stock picks…