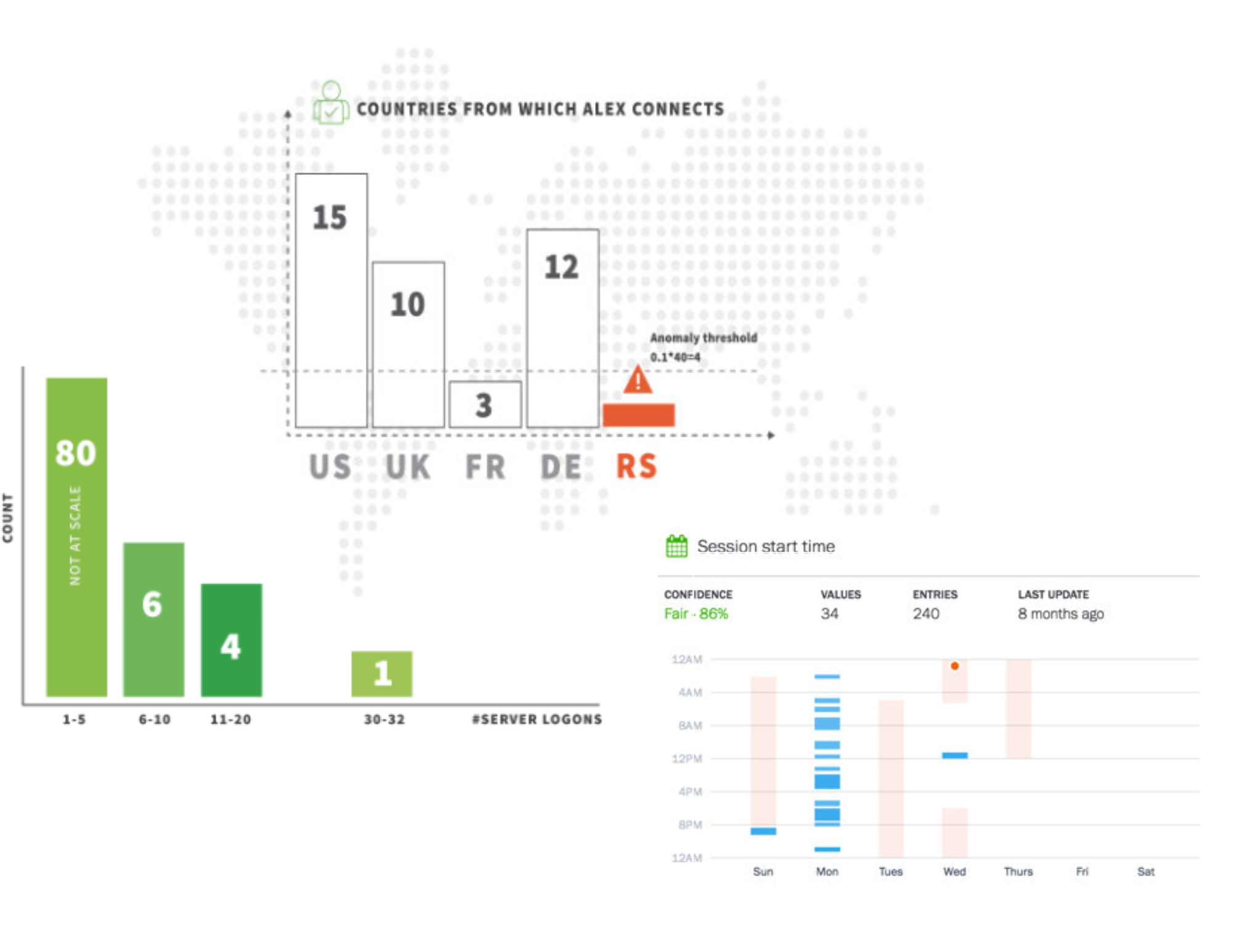
User and Entity Behavioural Analytics systems have changed the way organisations do security monitoring and have been responsible for detecting and thwarting some of the most major potential security breaches in the last few years. A UEBA system is often the first thing an organisation reaches for after they have been breached and in many cases they can even reduce the cost of security monitoring whilst also making that monitoring significantly more effective. But how does a UEBA system actually work and what makes them so effective in the Information Security battle?
The debate about wether UEBA is a replacement for or an adjunct to the traditional SIEM is still raging. To properly understand UEBA you probably need to start by looking at SIEM and how that technology has developed since it was introduced some thirty years ago. SIEM tends to work by collecting security log data both from security devices and from IT systems such as Windows, database, the network, Linux etc. SIEM Stands for Security Information and Event Management and Security Operations (SOC) teams leverage the events part to generate near real-time alerts based upon single or correlated event patterns and there in lies both the strength and the weakness of traditional SIEMs. The technology works well at small scale and a new owner of a SIEM will spend much of their time configuring rules for when to fire an alert. As more and more data is collected however the alert load also goes up and pretty soon is unmanageable. Engineering teams or senior SOC analysts spend more of their time trying to tune the alert correlation rules whilst the SOC 1st and 2nd line teams drown under a rising tide of false positive alerts. To make matters worse, when something interesting is detected the work really begins as the team must try to piece together the timeline to understand what actually happened and traditional SIEMs do little to help in that respect.
UEBA changed all of that with a completely new approach. Instead of worrying about individual events it builds statistical models for each user and each entity (IT system). I say models, in the plural, because a UEBA system may build hundreds of models for each user or entity, depending upon the types of activity associated with them. Clearly doing this is much harder than simply generating near real-time alerts and requires considerably more processing than a SIEM is capable of and for that reason UEBA systems tend to be somewhat heavyweight in terms of their hardware requirements.
For each user (let’s not worry about entities for the moment) events are matched based upon username or similar and then are categorised against the activity. So a user badging into a physical security system in the morning will generate a badge access even which will be stored in a model for badge access against a specific building or even entrance. the model grows day bay day as the user badges in and models for time of day as well as location will be developed. For time of days these will normally be bucketed to allow a histogram to be built. If a user normally badges in to the same building Monday to Friday “Normal” behaviour for that user will easily be established. If the user comes into work on a Sunday then that would be an anomaly, just as it would if the user came into work an Hour early or late. Similarly if they work from a different office, perhaps in a different country, the time may be the same but the building would be an outlier in the model so a location anomaly would be detected.
In the same way, users access different IT systems or performing some different activity within a monitored application might also generate anomalies, as would sending an unusual amount of data out of the organisation or to an unusual recipient, accessing an unusual web domain or receiving or sending “unusual emails”. There is no limit to what you can model in a system providing you have the data and some UEBA platforms give you the opportunity to build your own custom models.
Where UEBA systems differ from one another is what happens next. We have identified anomalies, but they are just that, anomalies, they are not necessarily bad behaviour. Some vendors then overlay the UEBA system with an expert system, which looks for kill-chains or something similar. Some will analyse the anomalies in a graph database looking for patterns in relationships and some will simply assign risk to each anomaly and let you decide how to handle that. The challenge here is that a bad actor, perhaps using a legitimate account will be mixed into the system and everyone, bad and good will be generating anomalies all of the time. An expert system suffers from the same weaknesses as traditional SIEM in that you need to know in advance what you are looking for, though possibly on a more abstract level.
One of the challenges that UEBA systems have are that different types of users behave differently and some have a great deal more variety in their day the others. System administrators for instance may work shift systems and they are very likely to access many more systems than normal users – Their normal behaviour generates anomalies. UEBA systems often use context as a first step in dealing with this problem. Rather than looking just at individuals and measuring their activity against past trends it is also possible to model their peer groups.
Identifying peer groups can be done in a variety of different ways. Looking at Active Directory groups is often an import first step but this can be problematic as different organisations us AD Groups in different ways and deep nesting of groups is common. Often some of the most interesting ML in a UEBA system is involved in dynamic peer group detection. This turns the problem on its head by using similar behaviour to detect the peering in the first place. Groups of individuals in an organisation tend to act in a similar manner so peer group detection and modelling is an opportunity to handle corporate variance. Whilst this will help with the system administrator problem it still doesn’t deal with the wide variance of each individual. You still need to reduce the risk allocation to avoid them constantly being notable. One interesting approach is to use Bayesian Inference, to back-off risks scores for known system admins or other individuals who would otherwise generate a lot of risk. I’ll talk more about Bayes Theorem in a different article.
Another thing that UEBA systems bring to the party is timelines though this isn’t specific to UEBA, it does seem to be associated with UEBA based solutions at present. One of the most difficult challenges for a SOC team is assembling the timeline of a users behaviour including both good and possible bad activity. Some UEBA systems build this timeline as they go and link individual activity to each individual model to allow a SOC analyst to investigate behaviour and make informed decisions. If the timeline only contains anomalies then it is fundamentally flawed as it is the behaviour around that which produced the anomaly which often sheds light on the overall activity.
One particular problem with modelling user behaviour is switches of context and any system which cannot handle this will leave significant gaps in behavioural visibility. A user who ssh’s into a Linux server then su’s to root or another user risks the UEBA system generating events into a different users timeline or simply not recording those events at all. Unless a UEBA system can stitch together the users behaviour across such context switches the overall system will be incapable of detecting real attacks as context switching is a staple of any early stage breach. In a similar way, IT hosts may have multiple contexts. A server may have more than one IP address and workstation IP addresses are likely to change from time to time if they rely upon DHCP. A UEBA system needs to map these together, particularly as some log sources will include IP addresses and some will give host names.
This however creates another challenge for us. Organisations with multiple domains may have users who are the same person in multiple domains (users also have break-glass accounts or developer and operations accounts in a single domain) and IP addresses often overlap, duplication addresses and often whole networks across the organisation. A Good UEBA system needs to join together the ones which are the same item but find some way of distinguishing those which are genuinely different. Often this process is made more difficult, if not impossible by poor management systems in the users environment. CMDB’s are rarely complete or accurate and are often spread across many different technologies and the quality of data in Active Directory is often very poor and may vary from one subdomain to the next. The lack of consistency or standard in context management is a severely limiting factor for both UEBA and SIEM and a huge opportunity for bad actors.
UEBA is a huge step forward in security breach detection at an early stage. The added effectiveness it brings to the SOC makes them more efficient and effective and pressure is removed from SOC engineering teams who no longer need to constantly tune rules looking for more of what happened last week. Not all UEBA systems are equal however and some traditional SIEM vendors have tried, with varying degrees of success, to morph into UEBA based systems. some systems are even traditional alert based SIEMs under the hood. If you are looking for a UEBA system try asking your vendor how it handles some of these challenges. If nothing else it will be a good indication of how those selling the product actually understand it.